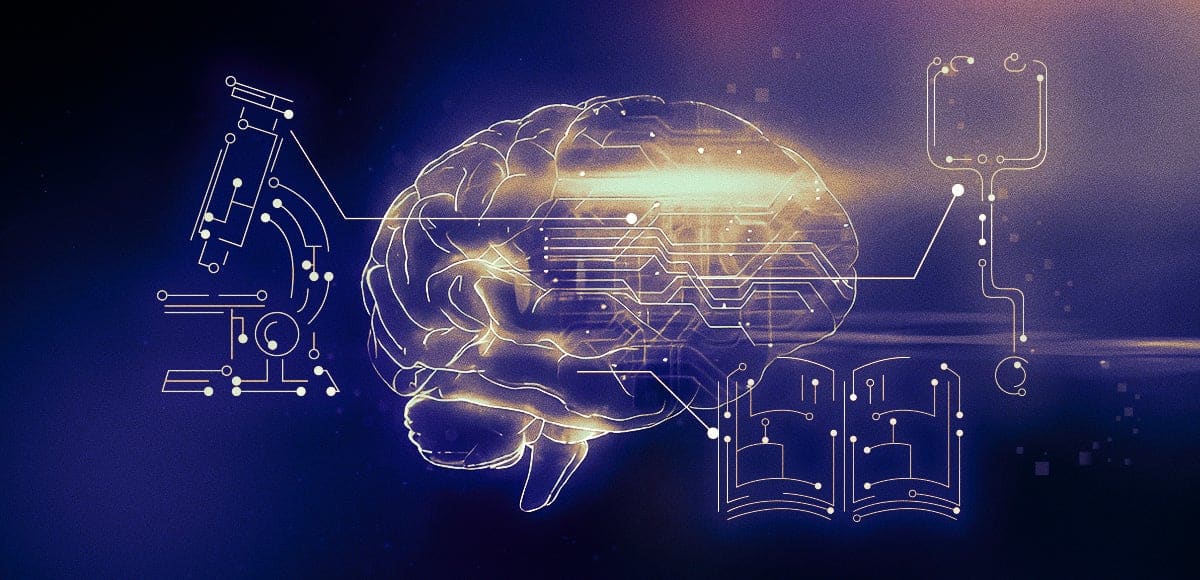
Governance by Algorithm: The Future of Decision-Making or a Recipe for Disaster?
Introduction
In recent years, the integration of algorithmic systems into governance has increasingly moved from the realm of science fiction into the practical and policy-making spheres. This shift has ignited a heated debate: are algorithms the future of decision-making, promising efficiency and fairness, or do they represent a perilous departure from traditional governance methods, fraught with risks and ethical dilemmas? This article explores the multifaceted implications of algorithmic governance, examining both its potential benefits and the serious challenges it presents.
- Understanding Algorithmic Governance
Definition and Scope
Algorithmic governance refers to the use of algorithms—sets of rules or procedures for solving problems or performing tasks—to make decisions typically handled by human administrators. These decisions could range from public policy and law enforcement to resource allocation and social services. Algorithms are used to analyse large datasets, identify patterns, and generate recommendations or decisions based on these analyses.
Types of Algorithms in Governance
- Predictive Algorithms: These forecast future events based on historical data, such as predicting crime hotspots or identifying which social services are needed in certain areas.
- Prescriptive Algorithms: These recommend specific actions based on data inputs, like suggesting policy changes or optimising public transportation routes.
- Descriptive Algorithms: These provide insights into current or past states, such as summarising the impact of recent policies or reporting on public health trends.
- Advantages of Algorithmic Governance
Efficiency and Speed
One of the most lauded benefits of algorithmic governance is its ability to process information rapidly and efficiently. Unlike human decision-makers, algorithms can handle vast quantities of data with speed and precision. This capability can lead to more timely responses to issues, such as addressing traffic congestion with real-time adjustments to traffic lights or distributing emergency services more effectively during a crisis.
Data-Driven Decisions
Algorithms offer the promise of data-driven decision-making, which can enhance objectivity and consistency. By relying on empirical evidence rather than subjective judgement, algorithmic systems aim to reduce bias and ensure that decisions are grounded in quantitative analysis. For instance, in resource allocation, algorithms can analyse patterns and needs more systematically than human administrators, potentially leading to more equitable distribution of resources.
Scalability
Algorithms can be scaled up to manage large and complex systems that would be unmanageable through traditional methods. In urban planning, for example, algorithms can analyse traffic flows, environmental impacts, and population growth simultaneously, providing insights that inform large-scale infrastructure projects. This scalability makes it possible to manage and optimise systems at a scale and complexity that would be challenging for human governance alone.
- Challenges and Risks of Algorithmic Governance
Lack of Transparency
A significant challenge with algorithmic governance is the opacity of many algorithms. Often, the workings of an algorithm—its logic, the data it uses, and the decision-making process—are not easily understandable to the general public or even to those implementing the algorithms. This lack of transparency can erode trust in the system and complicate accountability. When outcomes are unfavourable or decisions are contested, it can be difficult to pinpoint where the fault lies.
Bias and Discrimination
Algorithms are only as unbiased as the data they are trained on. If historical data reflects existing biases—whether racial, socio-economic, or otherwise—the algorithms can perpetuate and even exacerbate these biases. For example, predictive policing algorithms that rely on past arrest records might disproportionately target minority communities, reinforcing systemic inequalities. Thus, while algorithms can offer a veneer of objectivity, they may inadvertently entrench existing prejudices.
Dependence on Data Quality
The effectiveness of algorithms hinges on the quality of the data they process. Poor-quality, incomplete, or inaccurate data can lead to flawed or misleading outcomes. For instance, if a healthcare algorithm is fed with incomplete patient records, its recommendations could be harmful or suboptimal. Ensuring data integrity and accuracy is a critical challenge in algorithmic governance.
Accountability and Responsibility
Algorithmic decision-making raises complex questions about accountability. When an algorithm makes a decision that results in harm or injustice, determining who is responsible—whether it be the developers, the administrators who implemented the system, or the algorithm itself—can be problematic. This challenge necessitates clear frameworks for accountability and mechanisms for addressing grievances.
- Ethical and Societal Implications
Privacy Concerns
The use of algorithms in governance often involves the collection and analysis of large amounts of personal data. This raises significant privacy concerns, as individuals may be subjected to surveillance or have their data used in ways they did not anticipate or consent to. Balancing the benefits of data-driven governance with the protection of individual privacy is a key ethical consideration.
Human Autonomy
Algorithmic decision-making can potentially undermine human autonomy by delegating critical decisions to machines. This shift might affect individuals’ ability to have a say in decisions that impact their lives, such as the allocation of social services or the imposition of legal sanctions. Ensuring that human oversight remains a core component of algorithmic systems is essential to preserving individual agency.
Impact on Employment
The integration of algorithms into governance and administration can impact employment, particularly in roles that involve routine decision-making or data processing. While this could lead to increased efficiency, it also raises concerns about job displacement and the need for reskilling workers who may be affected by these technological changes.
- Case Studies and Real-World Examples
Smart Cities
Cities around the world are experimenting with smart city technologies that leverage algorithms for various purposes, such as traffic management, energy optimisation, and public safety. For example, Singapore uses an extensive network of sensors and algorithms to manage traffic flow and monitor air quality. While these systems offer improved efficiency and responsiveness, they also raise concerns about data privacy and surveillance.
Predictive Policing
Predictive policing uses algorithms to forecast where crimes are likely to occur and allocate resources accordingly. The Los Angeles Police Department’s use of predictive policing has led to increased efficiency in deploying officers, but it has also faced criticism for reinforcing existing biases and contributing to over-policing in certain communities.
Healthcare Algorithms
In healthcare, algorithms are used to diagnose diseases, recommend treatments, and manage patient care. While these tools can improve diagnostic accuracy and optimise treatment plans, they also face scrutiny regarding data privacy, the risk of reinforcing biases in medical care, and the need for human oversight in critical health decisions.
- Regulatory and Policy Considerations
Developing Standards and Guidelines
To address the challenges of algorithmic governance, it is crucial to develop comprehensive standards and guidelines. These should cover aspects such as transparency, fairness, data privacy, and accountability. Governments and organisations need to collaborate to establish frameworks that ensure algorithms are used responsibly and ethically.
Promoting Transparency and Accountability
Increasing transparency in algorithmic decision-making processes is essential to build public trust. This includes disclosing the data used, the criteria for decision-making, and the potential biases of the algorithms. Additionally, establishing clear lines of accountability and mechanisms for redress is vital for addressing grievances and ensuring fair outcomes.
Ensuring Inclusivity
Algorithmic systems must be designed to be inclusive and equitable. This involves actively addressing biases in data and algorithms, engaging with diverse stakeholders in the development process, and ensuring that the benefits of algorithmic governance are distributed fairly across different segments of society.
Conclusion
Governance by algorithm represents a profound shift in how decisions are made and executed in modern societies. While it offers significant advantages in terms of efficiency, scalability, and data-driven insights, it also presents substantial risks and challenges. The potential for bias, lack of transparency, and the impact on human autonomy and privacy are critical concerns that must be addressed through careful design, regulation, and oversight.
As we move forward, the balance between harnessing the benefits of algorithmic governance and mitigating its risks will be crucial. A thoughtful and inclusive approach to developing and implementing algorithmic systems can help ensure that these technologies serve to enhance rather than undermine the principles of good governance and democratic accountability.
References
Ziewitz, M. (2016). Governing Algorithms: Myth, Mess, and Methods. Science, Technology, & Human Values.
Zweig, K. A., Wenzelburger, G., & Krafft, T. D. (2018). On Chances and Risks of Security Related Algorithmic Decision Making Systems. European Journal for Security Research.